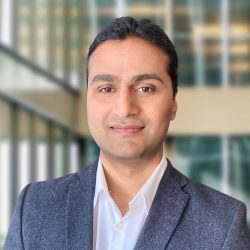
Ajit Desai
Principal Data Scientist
- Ph.D. Carleton University
- M.S. IIT Madras
Bio
Ajit Desai is a principal data scientist in the research division of the Bank of Canada's Banking and Payments Department. His current work leverages cutting edge techniques such as artificial intelligence, machine learning, and quantum computing to study payments data (including cryptocurrency data) with the primary objective of making Canada’s digital payments infrastructure safe and efficient. Dr. Desai received his Ph.D. from Carleton University in 2018 in Computational Science and Engineering and M.S. from Indian Institute of Technology Madras in 2011 in Aerospace Engineering.
Staff analytical notes
Staff working papers
Finding a Needle in a Haystack: A Machine Learning Framework for Anomaly Detection in Payment Systems
Our layered machine learning framework can enhance real-time transaction monitoring in high-value payment systems, which are a central piece of a country’s financial infrastructure. When tested on data from Canadian payment systems, it demonstrated potential for accurately identifying anomalous transactions. This framework could help improve cyber and operational resilience of payment systems.From LVTS to Lynx: Quantitative Assessment of Payment System Transition
We quantitatively assess the changes in participants’ payment behaviour from modernizing Canada's high-value payments system to Lynx. Our analysis suggests that Lynx's liquidity-saving mechanism encourages liquidity pooling and early payments submission, resulting in improved efficiency for participants but with slightly increased payment delays.Improving the Efficiency of Payments Systems Using Quantum Computing
We develop an algorithm and run it on a hybrid quantum annealing solver to find an ordering of payments that reduces the amount of system liquidity necessary without substantially increasing payment delays.Macroeconomic Predictions Using Payments Data and Machine Learning
We demonstrate the usefulness of payment systems data and machine learning models for macroeconomic predictions and provide a set of econometric tools to overcome associated challenges.Estimating Policy Functions in Payments Systems Using Reinforcement Learning
We demonstrate the ability of reinforcement learning techniques to estimate the best-response functions of banks participating in high-value payments systems—a real-world strategic game of incomplete information.Using Payments Data to Nowcast Macroeconomic Variables During the Onset of COVID-19
We use retail payment data in conjunction with machine learning techniques to predict the effects of COVID-19 on the Canadian economy in near-real time. Our model yields a significant increase in macroeconomic prediction accuracy over a linear benchmark model.Journal publications
Refereed journals
- Castro, P. S., Desai, A., Du, H., Garratt, R., & Rivadeneyra, F. (2024). Estimating policy functions in payment systems using reinforcement learning. ACM Transactions on Economics and Computation.
- McMahon, C., McGillivray, D., Desai, A., Rivadeneyra, F., Lam, J., Lo, T., Marsden, D. and Skavysh, V. (2024). Improving the efficiency of payments systems using quantum computing. Management Science.
- Chapman, J. T., & Desai, A. (2023). Macroeconomic predictions using payments data and machine learning. Forecasting, 5(4), 652-683.
- Desai, A., Lu, Z., Rodrigo, H., Sharples, J., Tian, P., & Zhang, N. (2023). From LVTS to Lynx: Quantitative assessment of payment system transition in Canada. Journal of Payments Strategy & Systems, 17(3), 291-314.
- Chapman, J. T., & Desai, A. (2020). Using payments data to nowcast macroeconomic variables during the onset of COVID-19. Journal of Financial Market Infrastructures, 9(1).
- Desai, A., Khalil, M., Pettit, C. L., Poirel, D., & Sarkar, A. (2020). Domain Decomposition of Stochastic PDEs: Development of Probabilistic Wirebasket-based Two-level Preconditioners. Journal of Computational Physics (review).
- Desai, A., Khalil, M., Pettit, C., Poirel, D., & Sarkar, A. (2018). Scalable domain decomposition solvers for stochastic PDEs in high performance computing. Computer Methods in Applied Mechanics and Engineering, 335, 194-222.
- Desai, A., Witteveen, J. A., & Sarkar, S. (2013). Uncertainty quantification of a nonlinear aeroelastic system using polynomial chaos expansion with constant phase interpolation. Journal of Vibration and Acoustics, 135(5).
- Desai, A., & Sarkar, S. (2010). Analysis of a nonlinear aeroelastic system with parametric uncertainties using polynomial chaos expansion. Mathematical Problems in Engineering.